Every click, login, and data packet exchanged across networks represents a potential entry point for malicious actors. Cyber threats evolve faster than ever before, adapting to bypass traditional firewalls and outdated protocols. To meet this growing challenge, artificial intelligence is quietly transforming the way systems detect, respond to, and neutralize risks—often before users realize an attack was even attempted.
If you’re looking for a short break from technical reading, this website offers engaging entertainment during downtime: this website features a variety of slots and games for quick, enjoyable distraction before diving back into complex topics.
AI-Powered Defense: Moving Beyond Traditional Firewalls
Manual threat detection methods rely heavily on signature-based systems. These systems identify threats by comparing incoming data to a known database of viruses or exploits. Unfortunately, this approach falls short when attackers modify tactics or create previously unseen malware.
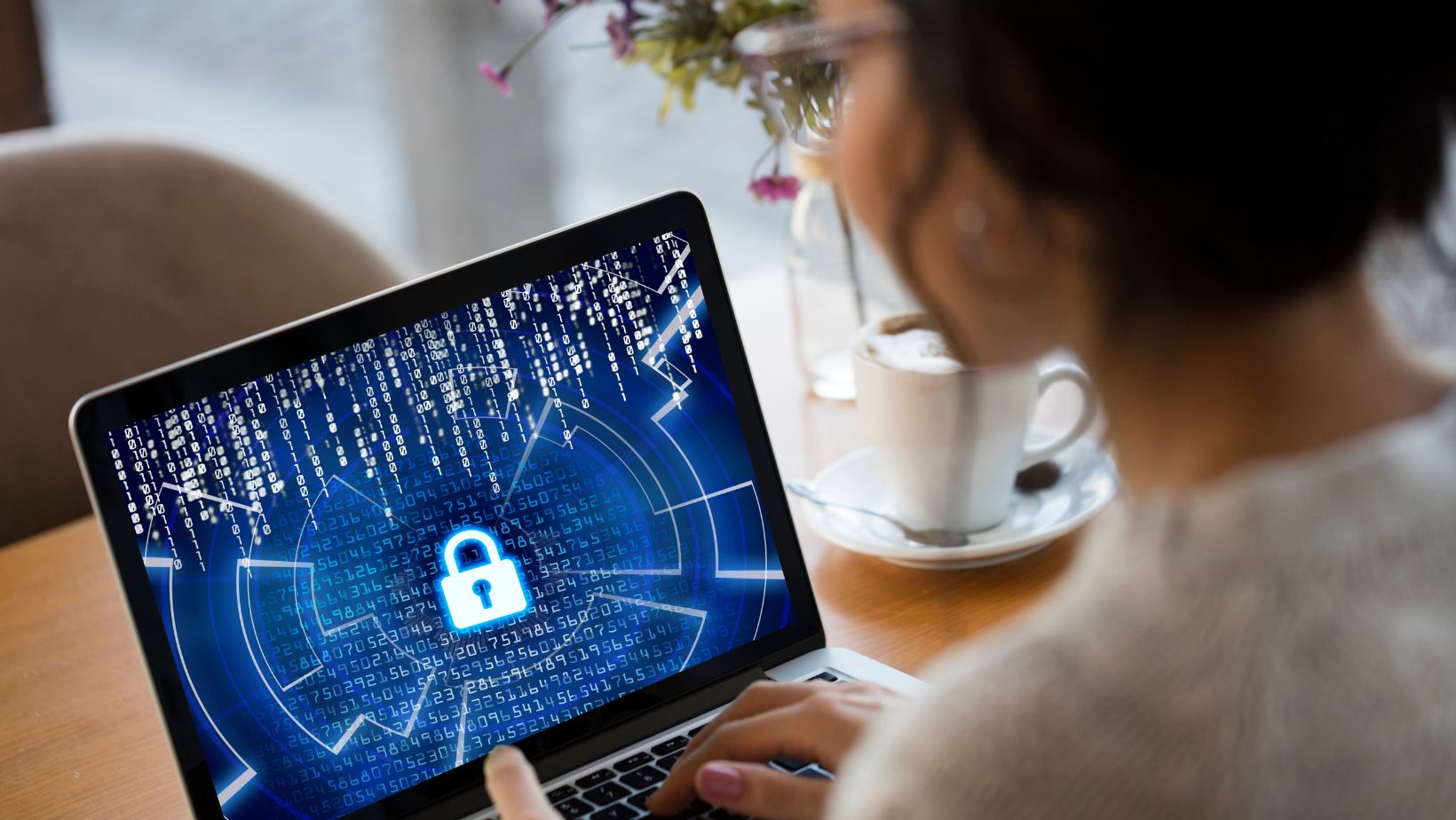
Artificial intelligence introduces dynamic learning mechanisms that detect patterns, not just known signatures. By analyzing user behavior, login frequency, access points, and other variables, machine learning algorithms can flag unusual activity almost immediately. This shift from reactive to proactive monitoring means that potential breaches can be intercepted before data is compromised.
Real-Time Threat Detection Using Machine Learning
AI models are capable of handling immense volumes of information with impressive accuracy. They continuously process activity logs, server behavior, and network traffic to establish baselines. Once these patterns are understood, deviations—no matter how subtle—are flagged for investigation.
How Algorithms Learn and Adapt
- Supervised Learning: Trained on labeled datasets that teach the system to recognize known types of attacks.
- Unsupervised Learning: Identifies anomalies without prior examples by clustering patterns that deviate from the norm.
- Reinforcement Learning: Uses trial-and-error to improve detection capabilities over time, reacting to feedback loops within the system.
Each method provides a layer of insight into suspicious activity, allowing security platforms to evolve alongside cyber threats.
AI in Identity Verification and Access Control
Safeguarding user credentials has never been more complex. Weak passwords, phishing campaigns, and credential stuffing can give unauthorized users access to sensitive systems. AI improves access management through biometric authentication, behavioral analysis, and real-time monitoring.
For instance, if a login attempt comes from an unusual location or device, the system may require multifactor verification or temporarily block the attempt. This form of adaptive access management reduces the risk of successful brute force attacks or session hijacking.
Cyber Threat Hunting with AI Assistants
While automated systems handle most threat detection, human analysts still play a vital role. AI doesn’t replace cybersecurity experts—it enhances their efficiency. By filtering out false positives and highlighting high-risk areas, intelligent tools allow analysts to focus on genuine threats.

Some common features in AI-assisted threat hunting tools include:
- Prioritized alerts based on severity and impact
- Contextual insights about threat origin, target, and potential damage
- Predictive analytics that estimate likely future attack vectors
These features turn raw data into actionable intelligence, empowering teams to make informed decisions quickly.
Ethics and Challenges of AI in Cyber Defense
With great power comes complex questions. AI-driven systems can be opaque, leaving users unsure why certain actions were taken. Biases in training data can also result in misclassification or missed threats.
Key concerns include:
- Transparency: How decisions are made needs to be understandable to non-technical users.
- Privacy: AI must monitor data without compromising personal information.
- Accountability: Systems should offer audit trails to clarify automated actions during security reviews.
Balancing speed, accuracy, and fairness remains a significant challenge as more companies integrate machine learning into their defense strategies.
The Future of AI in Cybersecurity
Looking ahead, the fusion of AI and cybersecurity will become even more seamless. Emerging fields like quantum computing and generative AI could either strengthen security or introduce new vulnerabilities. Preparing for these possibilities means designing systems that are not only intelligent but also adaptable and secure by default.
Trends to Watch:
- Increased use of Zero Trust Architecture supported by AI behavior models
- AI-assisted incident response automation to shorten downtime
- Collaboration between human analysts and AI agents in hybrid security operations centers
Organizations that embrace this synergy will be better equipped to fend off increasingly sophisticated attacks while protecting their users, assets, and reputations.